This project focuses on principles and methodologies relevant to the compression, storage and transmission of correlated sources, in particular from the viewpoint of sensor network applications. Research objectives include the derivation of theoretical source coding bounds relevant to these scenarios as well as the design of efficient compression, routing, and transmission algorithms, the latter objective particularly challenging due to the large-scale and non-convex optimization problems involved when considering real-world, many-sensor applications. Prediction, scalability, and robust design are but some of the issues, under the umbrella of distributed source coding, that are addressed by this project.
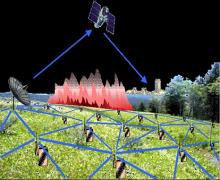
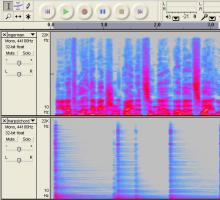
While audio and speech coding algorithms have historically developed via distinct compression paradigms - transform coding, and linear prediction - there is a growing impetus for a single algorithm that is efficient with either signal type, an integration necessitated by the increasing number of internet and mobile-based multimedia applications that handle 'mixed' content. This project focuses on developing unified coding paradigms for audio and speech signals, with the additional emphasis of ensuring performance that naturally scales with the availability of resources - bit-rate, encoding/decoding delay, and complexity - factors that considerably vary across the multitude of multimedia applications and devices that utilize these signals.
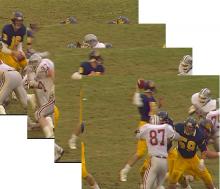
This research focuses on improving the performance of existing video coding algorithms by a number of novel techniques for predictive encoding or decoding as employed in these codecs. One thrust focuses on the optimal choice of the spatial transform that follows intra-prediction from block edges. Another direction being pursued is the development of estimation-theoretic algorithms for delayed decoding of video sequences encoded by motion compensation, wherein the reconstruction of the current video frame is improved by optimally utilizing information from 'future' frames of the sequence too.
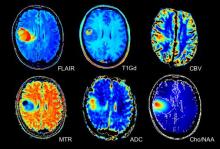
Imaging is at the core of many scientific discoveries, with information captured in terms of raw pixel intensities and in multiple channels for color or hyperspectral imagery. This project investigates the generation of probabilistic measurements and quantified uncertainties from image analysis methods, pattern classification methods to extract information that can be stored as probabilistic feature tables, and new approaches to visualization of probabilistic information, for the purpose of uncertainty management in the analysis of complex image data for scientific and engineering applications.
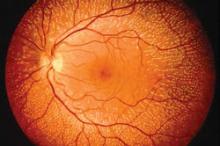
The critical barrier in the study of complex biological systems, for instance in the mapping of the vertebrate retina, are the computational challenges involved in analyzing the vast amount of images that are generated by such a project. The objective here is to address the lack of processing and discovery tools required to navigate data of such complexity and magnitude, and develop methodologies for large-scale, statistically robust, information integration, resulting in significant impact on image-based information processing technologies as well as neuroscience research.
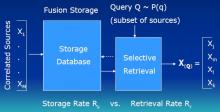
The objectives here are algorithms for efficient, interactive, and approximate or exact similarity search in high-dimensional data sets via approaches that draw inspiration not just from classical data management but also from several other disciplines including optimization, information theory, pattern recognition, and signal compression. The project eschews the perspective of treating the compression for database storage, and efficient retrieval problems separately, to demonstrate the myriad benefits of jointly optimizing both aspects.